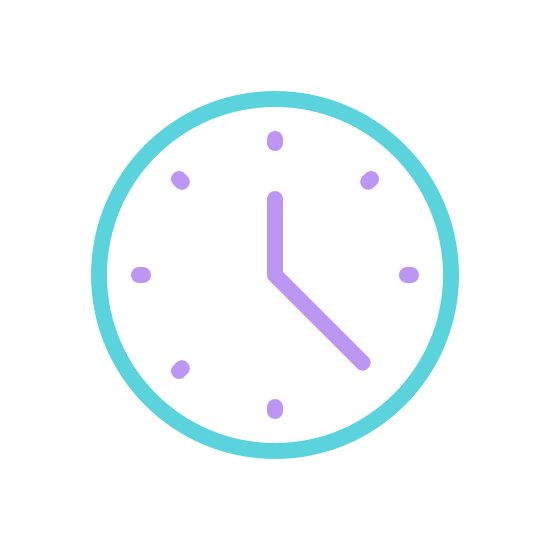
Have you ever hopped into your rideshare that was estimated to be a 13 minute ride only to be dropped at your destination 15 or more minutes beyond the initially communicated estimate? Uber and Lyft complete millions of trips per day in thousands of cities around the world and still routinely botch the estimates they surface to customers. How is that possible?
In an effort to find answers to this quandary, we decided to ask our very own David Vetrano. David is an Onfleet Co-founder, Chief Technology Officer and resident machine learning/computational neuroscience expert. He and his machine learning and data science teams at Onfleet have built the incredibly sophisticated predictive algorithms that provide the downstream estimates used by thousands of Onfleet customers in 90+ countries around the world.
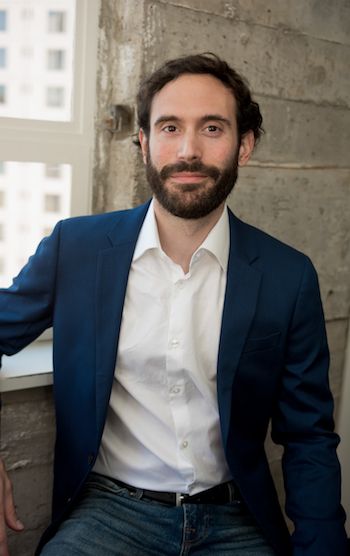
We started with this relatively simple question:
What's the hardest part of predicting the future?
Vetrano began by outlining how, at the core of this problem, it is incredibly hard to estimate how a car will move through our very unpredictable world. "Predicting future delivery arrival times requires us to update estimates very often as conditions change," says Vetrano. Onfleet uses novel machine learning based techniques along with up to 1,000 location data points per second to account for the rapidly changing environments that drivers are trying to navigate.
"We currently capture up to 1,000 locations per second and this number is growing" - David Vetrano, Co-Founder & CTO, Onfleet
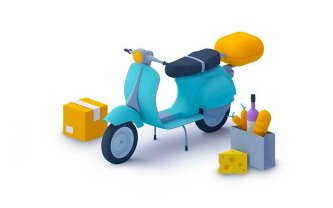
Traffic is an organic beast that can bottleneck streets for no predictable reason and predicting those "transit times on a road network is dependent on hyper-local location and precise time of day." This need for hyper-local location specific data is due to the fact that construction to fix a burst pipe comes with no calendar invite and that chihuahua running across the street definitely isn't looking both ways. Essentially, change is unpredictable, but up to the second, accurate information can offset this phenomena of change.
Onfleet's models account for inevitable fluctuations by processing our data as a whole from customers around the world. "Our models are constructed in a novel way to allow for hyper-local trends and to prevent interference between geographies while allowing for some insights to be leveraged across cities and regions." With companies operating in over 90 countries, these insights are incredibly robust.
"Our models are constructed in a novel way to allow for hyper-local trends and to prevent interference between geographies while allowing for some insights to be leveraged across cities and regions" - David Vetrano, Co-Founder & CTO, Onfleet

Many large companies, like the opening rideshare example, attempt to make predictions for their single type of delivery or business activity. On the contrary, Onfleet uses machine learning models to account for the plethora of delivery companies that rely on our predictive software to run their businesses around the world. Vetrano notes, "A large amount of time in the real world for deliveries is spent parking, reaching the destination, and on service time." These human factors are incredibly difficult to predict and can vary from industry to industry. To account for these variations Vetrano added, "We use separate models for these problems to leverage as much relevant data as possible for each problem while allowing for these kinds of differences across businesses and delivery models."
In the end, deliveries around the world and across industries can look very different. Predicting when these deliveries are going to be completed prior to the commencement of the actual delivery route requires the ability to dynamically adjust for changes within location based data. The more data that can be gathered and utilized, the better the estimates.
If you want to learn more about the machine learning models Onfleet utilizes to produce the most dynamic delivery estimates on the market, read the article Predicting the Future written by, you guessed it, David Vetrano.
Lastly, if you would like to learn how Onfleet might help your last-mile delivery operations, feel free to reach out here.